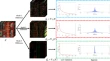
Overview
The journal publishes high-quality, peer-reviewed original papers, review papers and short communications on scientific computing.
- Editor-in-Chief
-
- Chi Wang Shu
- Impact factor
- 2.5 (2022)
- 5 year impact factor
- 2.8 (2022)
- Submission to first decision (median)
- 9 days
- Downloads
- 436,407 (2023)
Latest articles
Journal updates
-
Enhancing reproducibility of research papers in SISC, JSC and JCP
Hans De Sterck, Editor-in-Chief, SIAM Journal on Scientific Computing
Chi-Wang Shu, Editor-in-Chief, Journal of Scientific Computing
Rémi Abgrall, Editor-in-Chief, Journal of Computational PhysicsThis note is published simultaneously in SISC, JSC and JCP by the three editors-in-chief.
Reproducibility, that is, the ability to reproduce results obtained by others, is a core principle of the scientific method. In a concerted effort to enhance reproducibility in the area of scientific computing research, three of the leading journals in the scientific computing field will now invite authors, as part of the article submission process, to make the code and data publicly available that allow the results of their paper to be reproduced.
At article submission, the SIAM Journal on Scientific Computing (SISC), the Journal of Scientific Computing (JSC), and the Journal of Computational Physics (JCP) encourage authors to provide a link to their code and data files hosted in either a public git repository (e.g., on GitHub or Bitbucket), an open repository with DOI identification such as Zenodo or Mendeley Data, or journal supplementary materials.
At JCP and JSC, the link pointing to the code and data will be published prominently together with the paper. At SISC, authors may request a "SISC Reproducibility Badge: code and data available" which will be published with the paper as a recognition that the paper follows reproducibility principles.
While we acknowledge that it is not always possible for authors to make their code and data available, there are many good reasons to encourage authors to do so, including enhanced visibility and adoption of their work by the research community. We hope sincerely that many authors will take advantage of these new options to enhance and highlight the reproducibility of their scientific computing publications. -
Topical Collection: Beyond traditional AI: the impact of Machine Learning on Scientific Computing
Guest Editors:
Francesco Piccialli, francesco.piccialli@unina.it
Salvatore Cuomo, salvatore.cuomo@unina.it
Boumediene Hamzi, b.hamzi@imperial.ac.uk
Jan Hesthaven, Jan.Hesthaven@epfl.ch
Submission Deadline extended: February 28, 2022
We are pleased to solicit submissions to the Topical Collection "Beyond traditional AI: the impact of Machine Learning on Scientific Computing".
In order to add another piece to a complicated but fascinating puzzle, this topical collection aims to attract high-quality contributions to investigate both the role of ML/DL methodologies in applied mathematics and how Scientific Computing can benefit from learning paradigms. -
Topical Collection dedicated to the ICERM Spring 2020 semester program on Model Order Reduction
Guest Editors: Yanlai Chen, yanlai.chen@umassd.edu; Sigal Gottlieb, sigal_gottlieb@icerm.brown.edu; Serkan Gugercin, gugercin@vt.edu; Misha Kilmer, misha.kilmer@tufts.edu; Akil Narayan, akil@sci.utah.edu; and Daniele Venturi, venturi@ucsc.edu
Submission deadline: December 1, 2020
We are pleased to solicit submissions to the Topical Collection of JSC dedicated to the ICERM Spring 2020 program on "Model Order Reduction. Aiming to focus research effort on current areas of promising research and to galvanize new and existing collaborations, the Spring 2020 ICERM semester program focused on both theoretical investigation and practical algorithm development for reduction in the complexity - the dimension, the degrees of freedom, the data - arising in these models. The program in particular aimed to integrate diverse fields of mathematical analysis, statistical sciences, data and computer science, and specifically to attract researchers working in the areas of model order reduction, data-driven model calibration and simplification, computational approximation in high dimensions, and data-intensive uncertainty quantification. The four broad thrusts of the program are (1) mathematics of reduced order models, (2) algorithms for approximation and complexity reduction, (3) computational statistics and data-driven techniques, and (4) application-specific design.
Journal information
- Electronic ISSN
- 1573-7691
- Print ISSN
- 0885-7474
- Abstracted and indexed in
-
- ACM Digital Library
- BFI List
- Baidu
- CLOCKSS
- CNKI
- CNPIEC
- DBLP
- Dimensions
- EBSCO
- EI Compendex
- Google Scholar
- INSPEC
- INSPIRE
- Japanese Science and Technology Agency (JST)
- Mathematical Reviews
- Naver
- OCLC WorldCat Discovery Service
- Portico
- ProQuest
- SCImago
- SCOPUS
- Science Citation Index Expanded (SCIE)
- TD Net Discovery Service
- UGC-CARE List (India)
- Wanfang
- zbMATH
- Copyright information
-
© Springer Science+Business Media, LLC, part of Springer Nature